Research & Development
You can start from To Drive Innovation in.
Core Deep
Learning Research
2022
Present

Current and Expanding Frontiers (2022-Present)
- Experimentation with zero-shot learning and self-supervised learning..
- Development of Vision Language Models, bridging the gap between modalities. This is a requirement in one of our projects, so we had to make inroads in this area.
- Implementation of explainable AI (XAI) techniques, likely spurred by a growing emphasis on understanding and trustworthiness of AI models – specific requirements of Modern DL methods have to find any use in mission critical and health systems.
- We have explored and developed a few (continual / lifelong) training pipelines with Mixture of Experts (MoE) methods and Reinforcement Learning (RL) Hibernation.
- Research involving Bayesian Deep Learning, signifying deeper theoretical engagement, exploring Loss Landscapes and Deep Ensembles (One of our earlier deployments is a Deep Ensemble).
2020
2022

Evolution of Computer Vision Techniques and Search for Efficiency (2020-2022)
- Transformers made inroads into computer vision, and quickly achieved SOTA so we decided integration of Transformers and Attention Methods into our architectures.
- Focus on efficient training of vision models and engineering them for embedded systems.
- Exploration of ODENet – themselves and evolution of ResNet, and used them as backbones for various Computer Vision tasks.
2019
2020

Early Focus: Foundational Computer Vision Techniques (2019-2020)
- Initial work centered around object detection using CNNs (Convolutional Neural Networks). This is a foundational task in computer vision, suggesting earlier work in your timeline.
- Exploration of semantic and instance segmentation with CNNs. Segmentation further builds upon object detection and understanding.
2022
Present
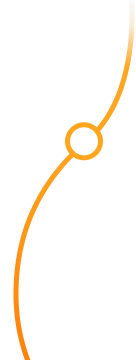
Current and Expanding Frontiers (2022-Present)
- Experimentation with zero-shot learning and self-supervised learning..
- Development of Vision Language Models, bridging the gap between modalities. This is a requirement in one of our projects, so we had to make inroads in this area.
- Implementation of explainable AI (XAI) techniques, likely spurred by a growing emphasis on understanding and trustworthiness of AI models – specific requirements of Modern DL methods have to find any use in mission critical and health systems.
- We have explored and developed a few (continual / lifelong) training pipelines with Mixture of Experts (MoE) methods and Reinforcement Learning (RL) Hibernation.
- Research involving Bayesian Deep Learning, signifying deeper theoretical engagement, exploring Loss Landscapes and Deep Ensembles (One of our earlier deployments is a Deep Ensemble).
Evolution of Computer Vision Techniques and Search for Efficiency (2020-2022)
- Transformers made inroads into computer vision, and quickly achieved SOTA so we decided integration of Transformers and Attention Methods into our architectures.
- Focus on efficient training of vision models and engineering them for embedded systems.
- Exploration of ODENet – themselves and evolution of ResNet, and used them as backbones for various Computer Vision tasks.
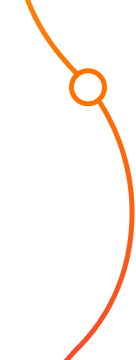
2020
2022
2019
2020

Early Focus: Foundational Computer Vision Techniques (2019-2020)
- Initial work centered around object detection using CNNs (Convolutional Neural Networks). This is a foundational task in computer vision, suggesting earlier work in your timeline.
- Exploration of semantic and instance segmentation with CNNs. Segmentation further builds upon object detection and understanding.
Applications
Core Technologies
- Facial Recognition & Human Gait Recognition: Classic computer vision problems used in core products.
- Scene Understanding & Description: Advanced tasks for creating structured and vector representations of scenes, enabling free-text and similarity queries.
- Action Recognition: Enhances scene understanding by identifying and interpreting human actions.
Innovations
- Multi-object Trackers (MOTs): Lightweight and accurate tracking solutions.
- Background Subtraction Methods: Proprietary techniques for use-cases like waste-dumping and intrusion detection.
- SOTA OCR Method: Combined with detection models for unique Automatic Number Plate Recognition (ANPR) systems.
- VMS Software: Employs novel image and video codecs and compression techniques to enhance performance and efficiency in various applications.
Proprietary Development
- Proprietary Architectures: Most in-house development is proprietary and unpublished. Plans to publish exist, but not at the cost of our competitive edge.
- Scene Understanding & Description: Advanced tasks for creating structured and vector representations of scenes, enabling free-text and similarity queries.
- In-house Capabilities: Strong focus on developing robust in-house R&D capabilities, despite leveraging global methods and research.
Software Development
- Critical Role: Software development teams are essential for transforming research into large-scale systems serving millions.
- Engineering Effort: Enormous engineering effort involving numerous tools, techniques, and processes to bring ideas to market.
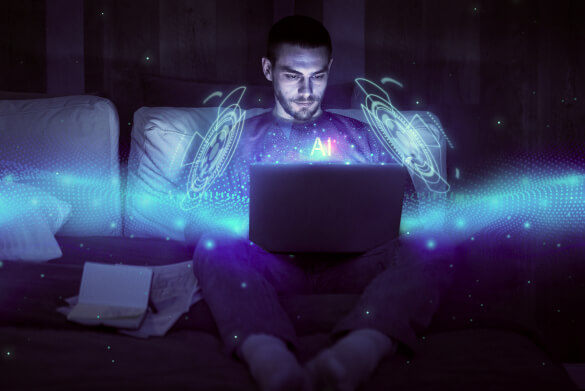
Focus of Research and Development in Trois
We believe research and development are crucial for driving innovation, maintaining competitiveness, meeting customer demands, and ensuring long-term growth and success within a product company.
Energy Efficient
Computing Devices
Computer Vision, Deep
Learning and AI
Drone Control
System
High Fidelity
Optical Systems
Energy Efficient
Communication Systems
Energy Efficient
Software
Ongoing Research
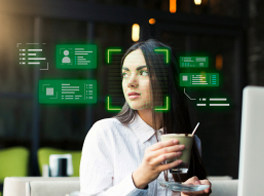
Face
Recognition
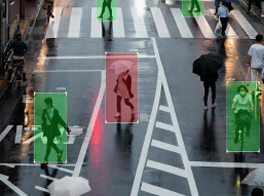
Gait
Recognition
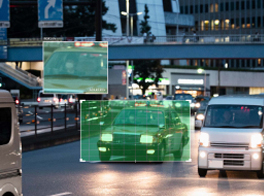
Automatic Number
Plate Recognition
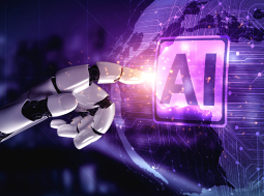
Generative Artificial
Intelligent
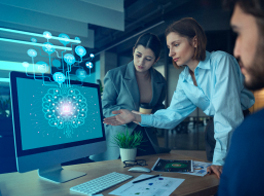
Explainable
Artificial Intelligent
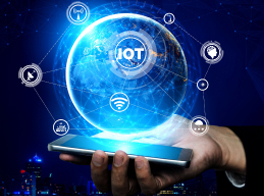
IoT Systems
and Platforms
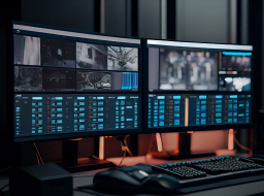
Video Management
System
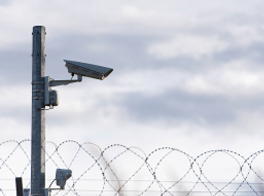
Perimeter Security
Systems
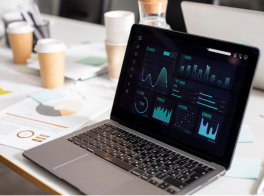
Video Analytics
Platform
Research & Development Collaborations
Crafting the Future with Shared Expertise and Innovation
To build products and solutions that performs ahead of the times, we have wisely chosen Public and private sector Institutions, Companies with Research and Development Capabilities.
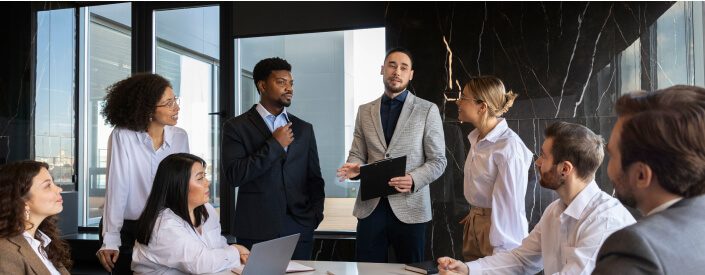
Shared Expertise
By bringing together experts to tap into each other's specialized knowledge and skills from different fields, industries, or research areas, collaborations can lead to a more comprehensive understanding of the problem at hand and generate novel solutions.
Resource Pooling
Collaborating entities can share the financial burden, laboratory facilities, equipment, and other resources required for research, making it more feasible to undertake ambitious projects that might have been challenging to pursue independently.
Risk Sharing
Innovation inherently involves some degree of risk. By collaborating, organizations can share the risks associated with R&D efforts, making it less daunting for each individual participant.
Access to New Markets and Applications
R&D collaborations can lead to the creation of innovative products or technologies that open up new market opportunities for the participating companies.
Faster Time-to-Market
With more minds working on a problem, R&D collaborations can expedite the development process, leading to quicker commercialization of new products or services.
Intellectual Property (IP) Management
Intellectual property rights are crucial in R&D collaborations. We always have clear agreements regarding the ownership and usage of any IP resulting from the collaboration.
Joint Publications and Recognition
Many R&D collaborations lead to significant findings and breakthroughs. Collaborating entities often publish joint research papers, which can enhance their reputation and credibility in their respective fields.
Long-Term Relationships
We believe Successful R&D collaborations can build long-term relationships between organizations, leading to future collaborative projects and partnerships.